The trucking industry faces significant challenges when it comes to safety. Every year, thousands of accidents involving trucks result in injuries, fatalities, and massive financial losses. While efforts to improve driver training and vehicle standards have been ongoing, one of the most promising tools to reduce accidents lies in artificial intelligence (AI) and machine learning (ML). These technologies are being increasingly adopted by fleet operators to predict and prevent accidents before they happen, enhancing overall road safety.
How AI and Machine Learning Work in Trucking
AI and machine learning are powerful tools that can analyze vast amounts of data to identify patterns and make predictions. In trucking, these systems are integrated into fleets to monitor various risk factors in real-time and offer actionable insights.
- Driver Behavior Monitoring: AI-powered systems can monitor driver behavior to detect unsafe driving practices like speeding, harsh braking, tailgating, or fatigue. By using cameras and sensors, these systems analyze how a driver operates the vehicle and alert them when their behavior indicates a higher risk of an accident. This real-time feedback can help drivers make adjustments on the road, potentially preventing dangerous situations.
- Predictive Maintenance: Machine learning algorithms can predict when parts of the truck are likely to fail, based on historical data from sensors installed in vehicles. For example, tire pressure, brake condition, and engine performance are continuously monitored, and the system alerts the fleet manager when maintenance is required before a breakdown occurs. This reduces the chance of mechanical failures that could lead to accidents.
- Fatigue Detection: One of the leading causes of trucking accidents is driver fatigue. AI can monitor driver alertness by analyzing facial expressions, eye movement, and even head position. These systems can then warn drivers when they are showing signs of drowsiness, prompting them to take a break before fatigue impairs their ability to drive safely.
The Role of Data in Predicting Accidents
AI and machine learning systems are heavily reliant on data. The more data they have, the better they can predict potential accidents. This includes:
- Telematics Data: Information from GPS systems, accelerometers, and vehicle sensors is continuously collected. AI processes this data to create driver profiles, identify risky behaviors, and forecast potential accident scenarios based on past incidents.
- Weather and Traffic Data: AI systems can integrate external data such as weather conditions and traffic patterns to predict how they might affect driving conditions. For example, if a storm is approaching, the system can alert drivers to slow down or reroute them to avoid dangerous conditions.
- Historical Accident Data: Machine learning models can be trained on historical accident data to identify the circumstances that lead to crashes. These models can then predict the likelihood of accidents occurring in similar situations, helping fleet managers take preemptive measures.
Real-World Applications and Results
Many trucking companies and logistics firms have already seen significant improvements in safety by adopting AI and machine learning solutions. For instance, fleets equipped with AI-driven driver monitoring systems have reported fewer incidents related to distracted driving, speeding, and fatigue.
- Reduced Accident Rates: AI and ML systems have been credited with reducing accident rates by providing early warnings and corrective feedback to drivers. One study reported that fleets using AI safety systems saw a reduction in accidents by as much as 30%.
- Lower Insurance Costs: Insurers are beginning to recognize the benefits of AI-driven accident prevention systems. Fleets that use AI technology are often eligible for lower insurance premiums due to their improved safety records.
- Enhanced Driver Training: The data collected by AI systems is also valuable for training purposes. Fleet managers can use real-world data to create personalized training programs that focus on specific weaknesses or unsafe driving habits, ultimately fostering a culture of safety.
Challenges and Limitations
While AI and machine learning have clear benefits, they are not without challenges. The initial investment in AI systems, including hardware like sensors and cameras, can be high. Additionally, there is a learning curve associated with using these technologies effectively, as fleet operators and drivers need to adapt to new systems.
Privacy concerns also arise, especially with driver monitoring. Some drivers may feel uncomfortable with constant surveillance, even if it’s for safety purposes. Companies need to strike a balance between enhancing safety and respecting privacy, ensuring that data collected is used responsibly.
The Future of AI in Trucking Safety
As AI and machine learning technologies continue to evolve, their potential to revolutionize trucking safety will only grow. Autonomous trucking, although still in development, could one day eliminate many of the risks associated with human error. Until then, AI and ML will continue to play a critical role in predicting and preventing accidents, helping to save lives and reduce costs for fleet operators.
By adopting these technologies, trucking companies can ensure safer roads, better performance, and a more efficient fleet. The future of trucking safety is not just in the hands of drivers but also in the algorithms designed to protect them.
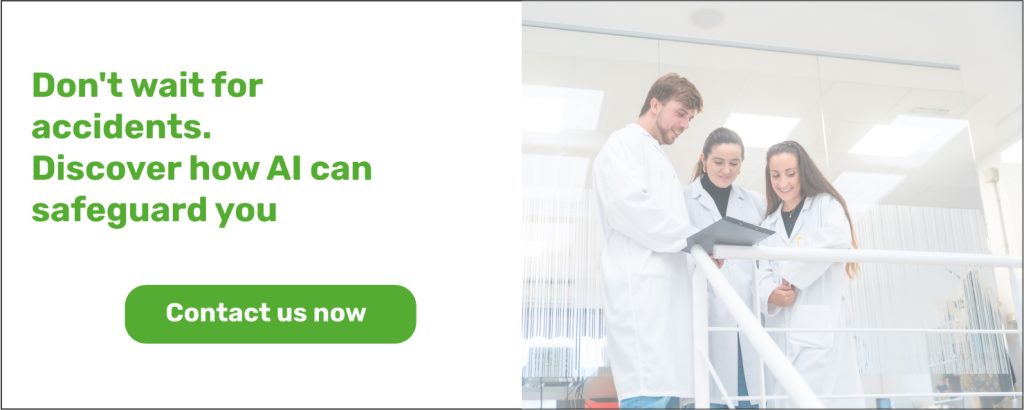